Navigating the World of Red and Green AI for Sustainable Development
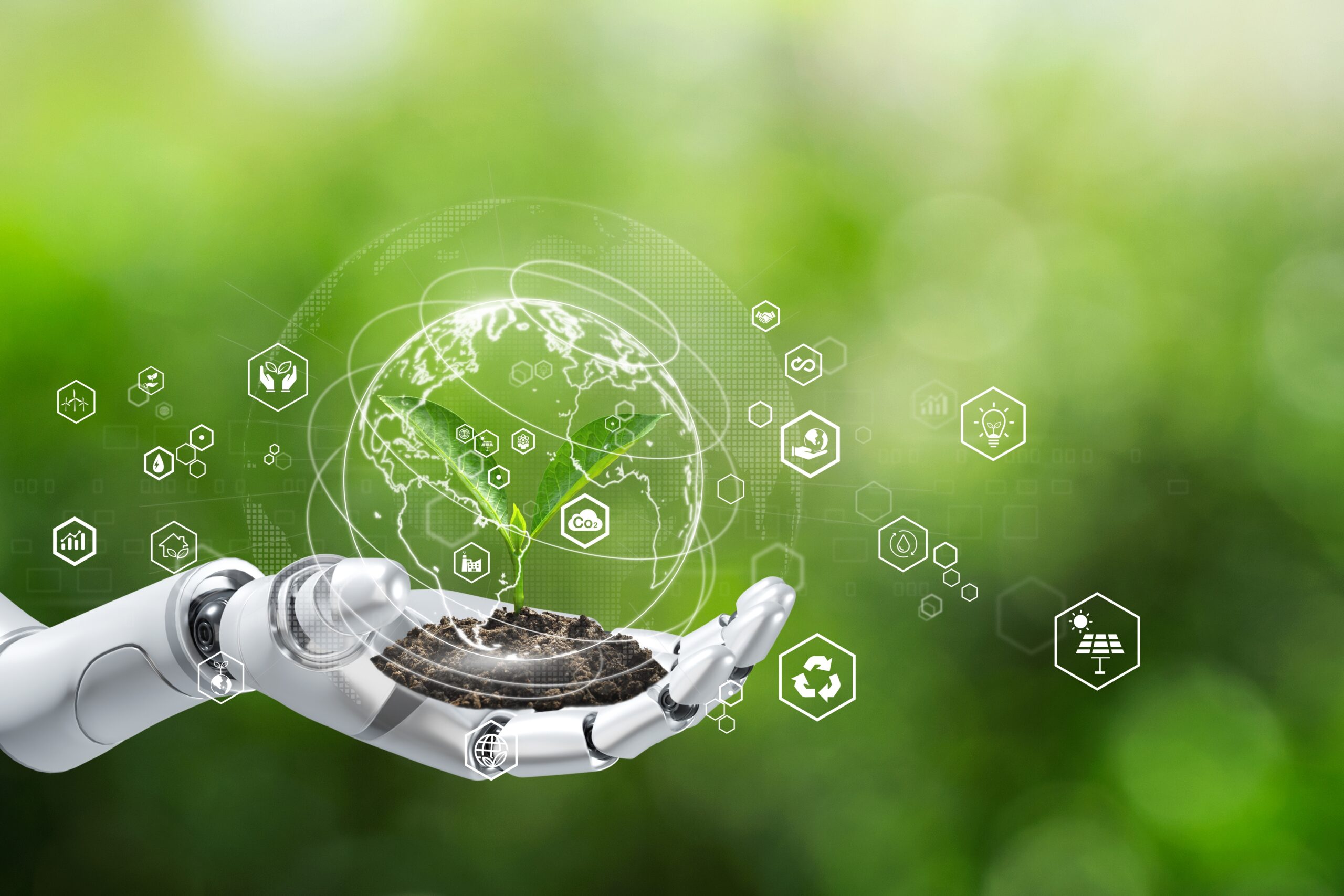
The increasing complexity of AI models has led to the development of two distinct approaches: green AI and red AI. This Tokenhell guide aims to provide a comprehensive understanding of these concepts.
Recently, the field of AI has witnessed a surge in the development of advanced models. This progression has had positive and negative environmental repercussions, giving rise to the concepts of red AI and green AI.
The environmental ramifications and carbon emissions associated with red AI have raised considerable concerns due to the extensive energy and computational resources required for training these advanced models.
On a brighter note, green AI offers an environmentally friendly alternative. This approach, championed by conscientious developers, strives to reduce the environmental burden imposed by the computational requirements of deep learning algorithms.
We will explore their definitions, characteristics, impacts, and applications to gain a deeper insight into green AI and red AI.
AI-Related Costs
The expenses linked to AI are diverse and originate from various sources. These costs encompass the computational resources for training AI models, the data necessary for ongoing maintenance, and the environmental impacts of significant energy use during operations. Key costs associated with AI include:
- Computational expense: Training AI models necessitates specific hardware infrastructure involving substantial financial investment. As AI models become more complex and seek higher accuracy, the cost of training escalates, encompassing the high energy expenses incurred in training complex AI models, which are energy-intensive.
- Carbon footprint: The ecological cost of AI is significantly characterized by its carbon footprint, mainly due to the extensive energy used in training advanced AI models.
- Social cost: Achieving fair and unbiased outcomes requires resources and efforts to identify and correct biases in AI algorithms.
- Maintenance and evolution costs: These costs arise from continuously monitoring and adapting AI systems, ensuring their proper functioning, and addressing any emerging issues. The ongoing need to update and adjust AI systems to keep up with changing needs, technological progress, and evolving standards also incurs costs.
Red AI
Red AI addresses the environmental challenges associated with artificial intelligence, focusing on the significant ecological impacts during the training phases of machine learning models, which demand considerable computational resources and energy consumption.
Red AI calls for measures to reduce its environmental footprint, advocating for optimized practices, a shift to renewable energy sources, and adopting sustainable methods. The central theme is acknowledging the environmental consequences of conventional AI practices and the need to transition to more sustainable methodologies and technologies. This encompasses the optimization of algorithms, the use of renewable energy, and efforts to minimize the ecological impact of AI systems.
Moreover, the environmental impact of red AI goes beyond carbon emissions, encompassing broader ecological concerns in AI development, such as electronic waste, water usage, and other environmental stress factors.
Green AI Concept
Green AI represents an evolution in artificial intelligence, striving to reduce emissions to negligible levels and address potential environmental concerns. This iteration of AI development is centered on sustainability, highlighting the importance of energy-efficient practices throughout the entire lifecycle of AI systems. It promotes the utilization of renewable energy sources in AI operations to decrease dependence on non-renewable energy.
By adopting these methodologies, green AI aims to lessen the environmental footprint of AI development, tackling issues such as carbon emissions, electronic waste, and overall resource consumption. Beyond mitigating environmental impacts, green AI significantly contributes to combating climate change by integrating sustainable practices in various sectors.
The commitment to green AI provides an avenue for artificial intelligence to mitigate environmental challenges and effectively address broader climate issues. This transition to sustainability positions AI as a key player in supporting sustainable practices in residential, urban, and industrial settings.
Areas of Green AI Application
Green AI finds its application in numerous sectors, enhancing sustainability and diminishing environmental impacts. Notable areas of impact include:
- Enhancing energy efficiency in data centers through AI algorithms that optimize operations reduces energy use in activities like cooling and server management.
- Improving smart grids and energy management by employing AI for predictive analysis enhances energy distribution efficiency and minimizes waste.
- Supporting climate modeling and forecasting, where green AI assists scientists and policymakers in understanding and addressing climate change more effectively.
- Aiding the transportation sector by reducing traffic congestion and refining logistics operations through AI solutions.
Progressing with Green AI
Advancing green AI necessitates a focus on algorithmic optimization. Developing and implementing algorithms that prioritize energy efficiency and reduce computational demands is essential, thereby lessening AI’s environmental impact and promoting the progression of green AI.
Fostering collaboration among researchers, organizations, and industry stakeholders is also vital. This collaboration encourages the exchange of best practices, insights, and developments in green AI, leading to a unified effort towards sustainability. Incentives like tax benefits or certifications for organizations that adopt and implement green AI practices can stimulate broader adoption.
Final Thoughts
Artificial intelligence has been making significant advancements in the tech industry. However, regarding sustainability, AI is still in its early stages of development. Addressing this requires identifying and employing the most effective systems, methods, and approaches to build powerful and sustainable AI models.
By adopting a suitable approach and seeking expert technology consultation, there is an opportunity to create AI tools that push the limits of what is possible and conform to environmental responsibility principles. As we explore the dynamics of green AI versus red AI, prioritizing sustainability is crucial for the ongoing evolution of AI.
Tokenhell produces content exposure for over 5,000 crypto companies and you can be one of them too! Contact at info@tokenhell.com if you have any questions. Cryptocurrencies are highly volatile, conduct your own research before making any investment decisions. Some of the posts on this website are guest posts or paid posts that are not written by Tokenhell authors (namely Crypto Cable , Sponsored Articles and Press Release content) and the views expressed in these types of posts do not reflect the views of this website. Tokenhell is not responsible for the content, accuracy, quality, advertising, products or any other content or banners (ad space) posted on the site. Read full terms and conditions / disclaimer.